For Scientists, by Scientists
Powered by industry-leading technologies developed at Mila, the world’s largest deep learning research institute
Pioneering few-shot learning for drug design
Novel methods built for low data environments are needed to unlock the full potential of deep learning in drug design.
Our expansive portfolio of technologies builds on our founding team’s pioneering work in few-shot learning for drug design, allowing us to learn accurate property predictors from datasets too small, sparse, or noisy for traditional computational methods.
Whether a novel target class with limited historical data, or low-throughput assays difficult to run at scale, Valence's technologies unlock new frontiers in AI-enabled drug design.
Improving the generalization and expressivity of graph neural networks
Machine learning on graph-structured data is an essential component of modem drug design workflows.
Our team is working to improve the expressivity and generalization capacity of graph neural networks, ensuring consistent model performance across different classes of molecules and targets.
This extends to the dynamic, three-dimensional nature of molecules, ensuring best-in-class performance on bioactivity prediction, ADME-Tox profiling, and graph-based molecular generation.
Unifying molecular design with real-world chemistry constraints
Effective drug design requires tradeoffs between property optimization and real-world chemistry constraints.
We put synthesizability first, developing technologies for molecular design that use measures of synthetic feasibility as a means of more efficiently searching global design space.
Whether constrained optimization or de novo design, we combine reinforcement learning, generative modeling, and active learning strategies to identify molecules optimized for multiple objectives, while also enforcing a high degree of medicinal chemistry quality at every step.
Latest Research
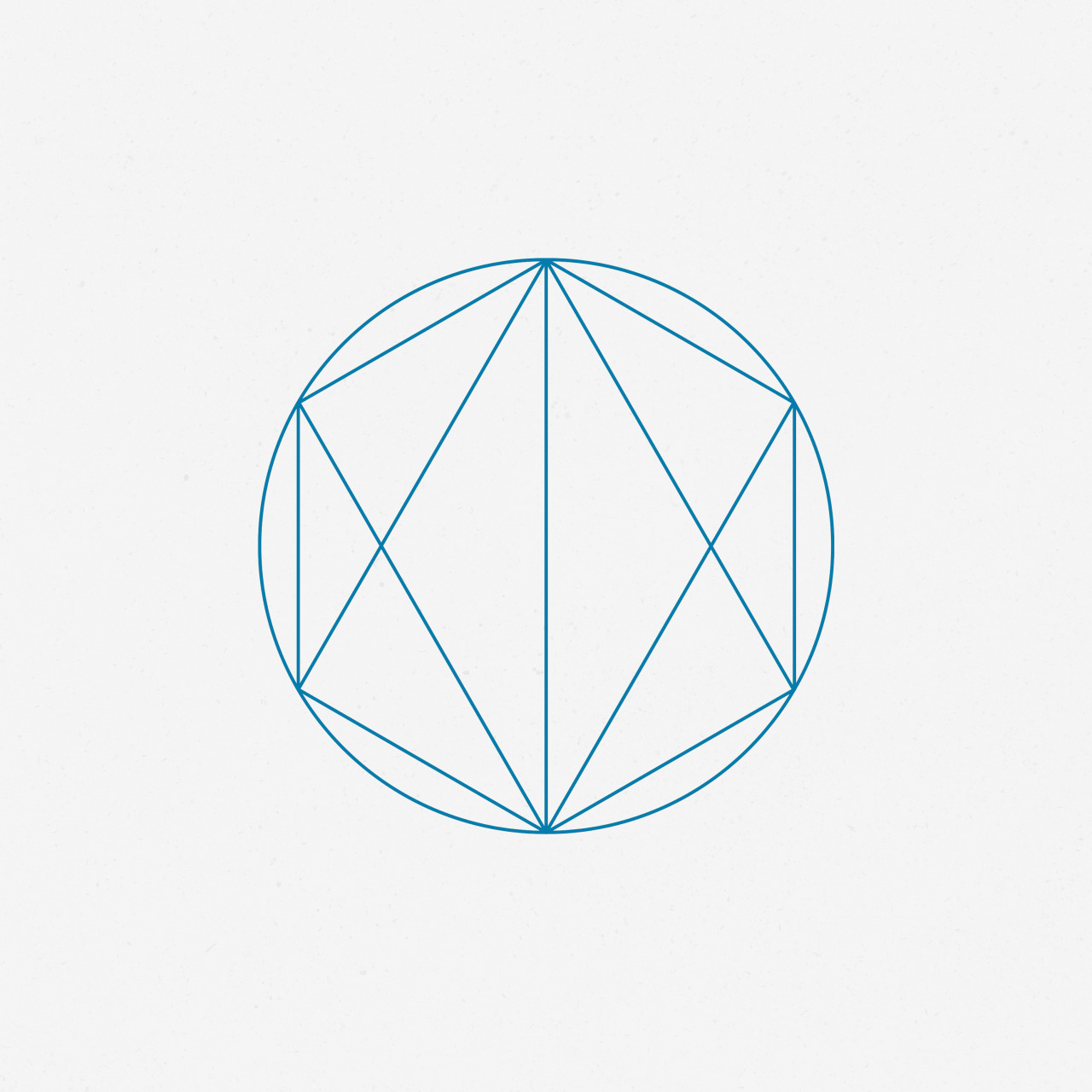
Long Range Graph Benchmark
Published in
NeurIPS
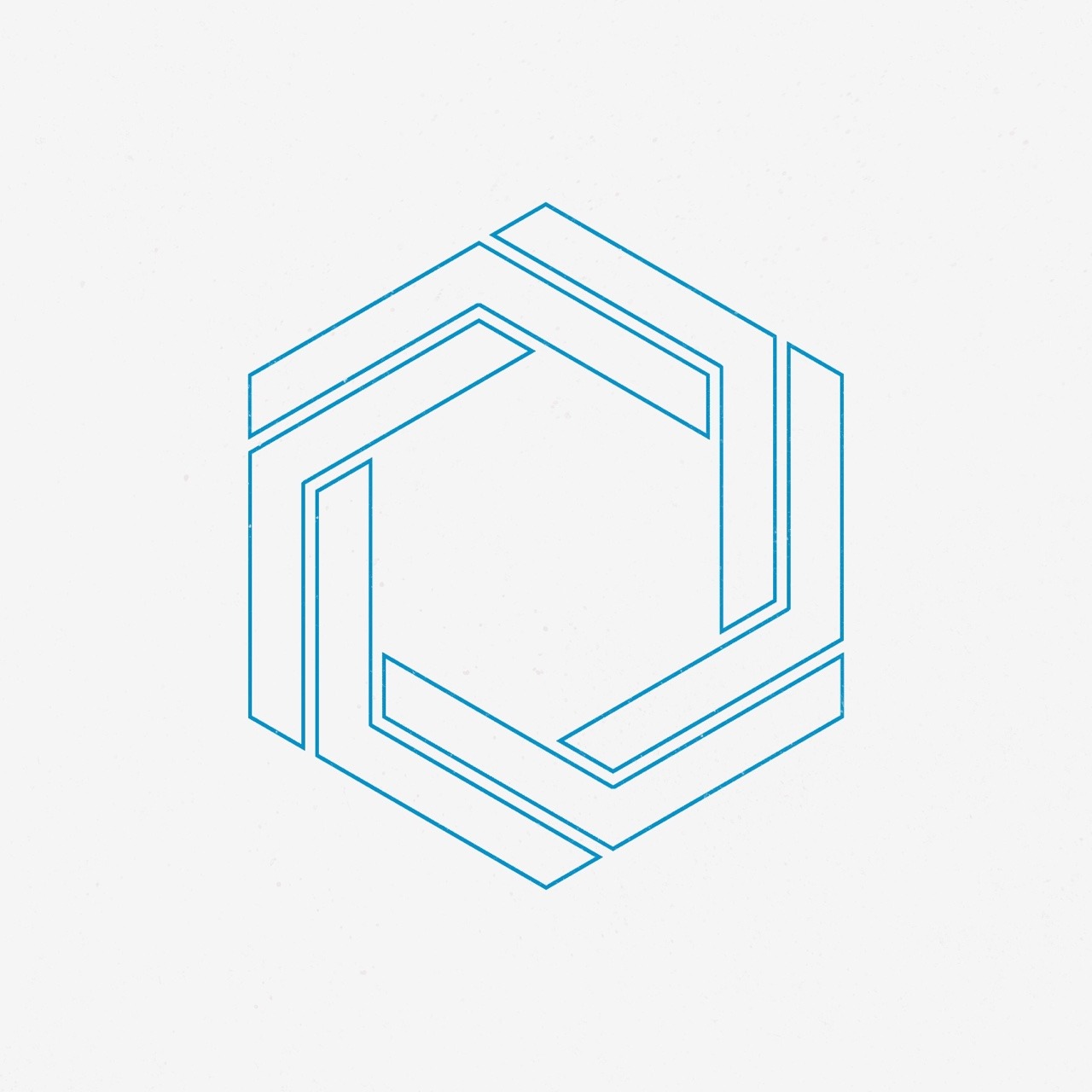
Recipe for a General, Powerful, Scalable Graph Transformer
Published in
NeurIPS
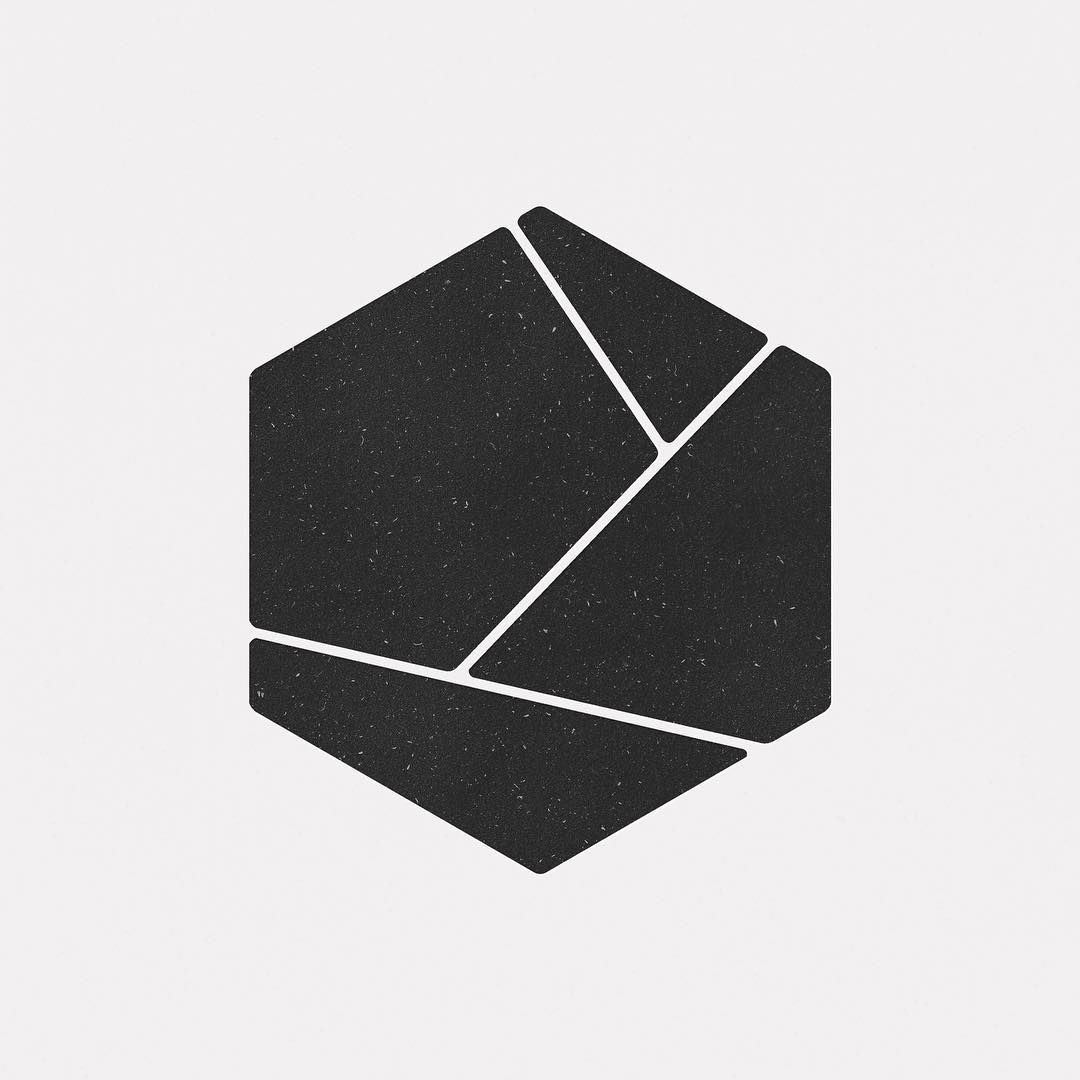
3D Infomax Improves GNNs for Molecular Property Prediction
Published in
arXiv
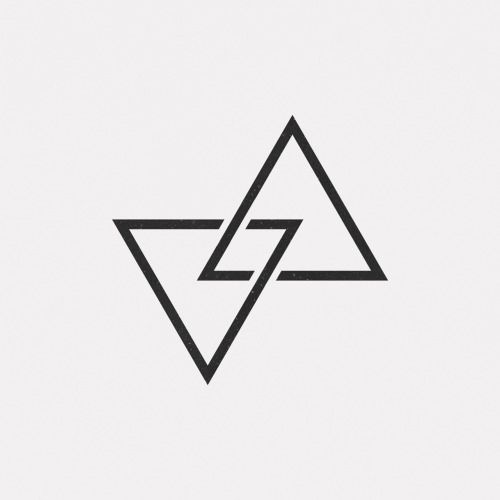
On the Robustness of Generalization of Drug–Drug Interaction Models
Published in
BMC Bioinformatics

Rethinking Graph Transformers with Spectral Attention
Published in
NeurIPS
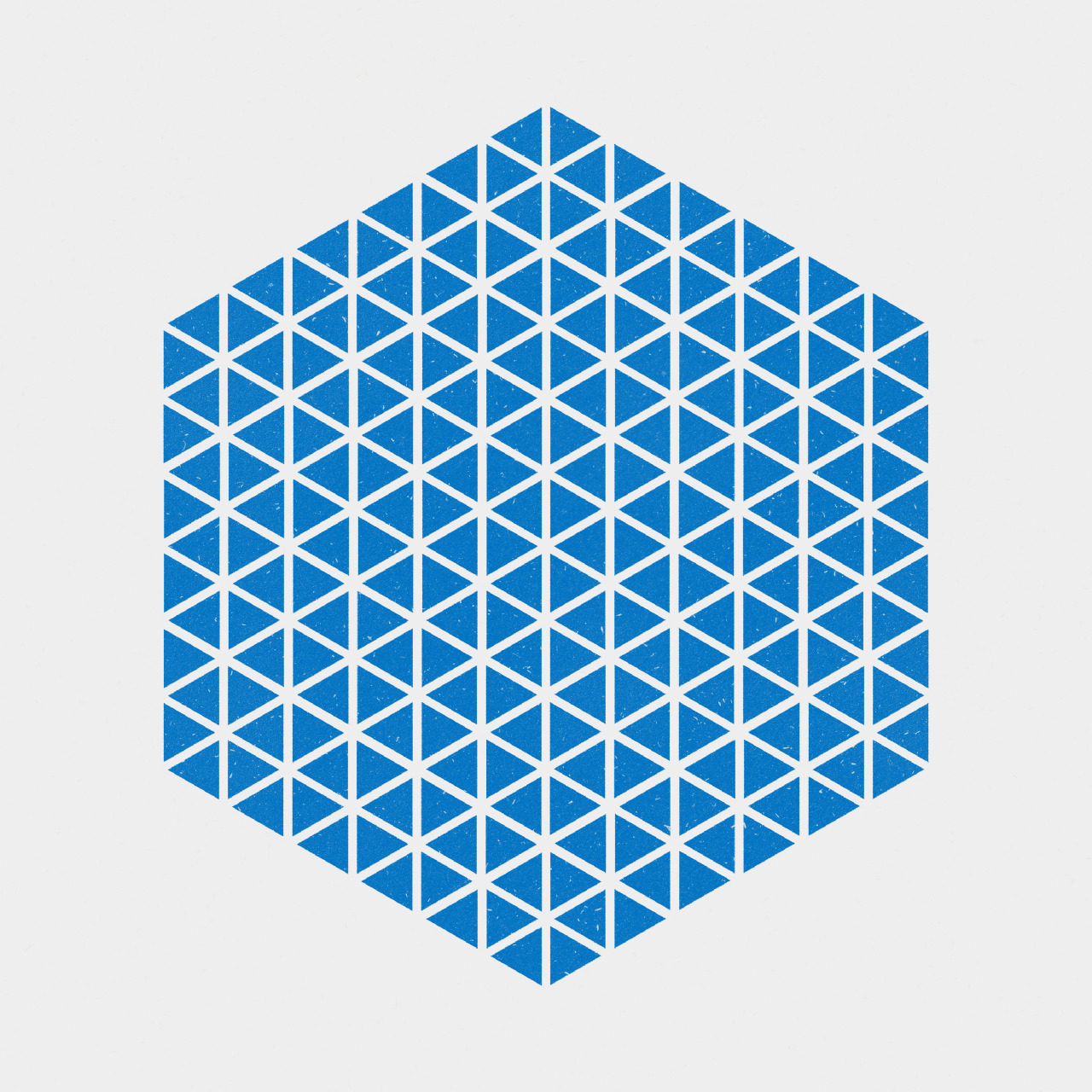
Directional Graph Networks
Published in
ICML
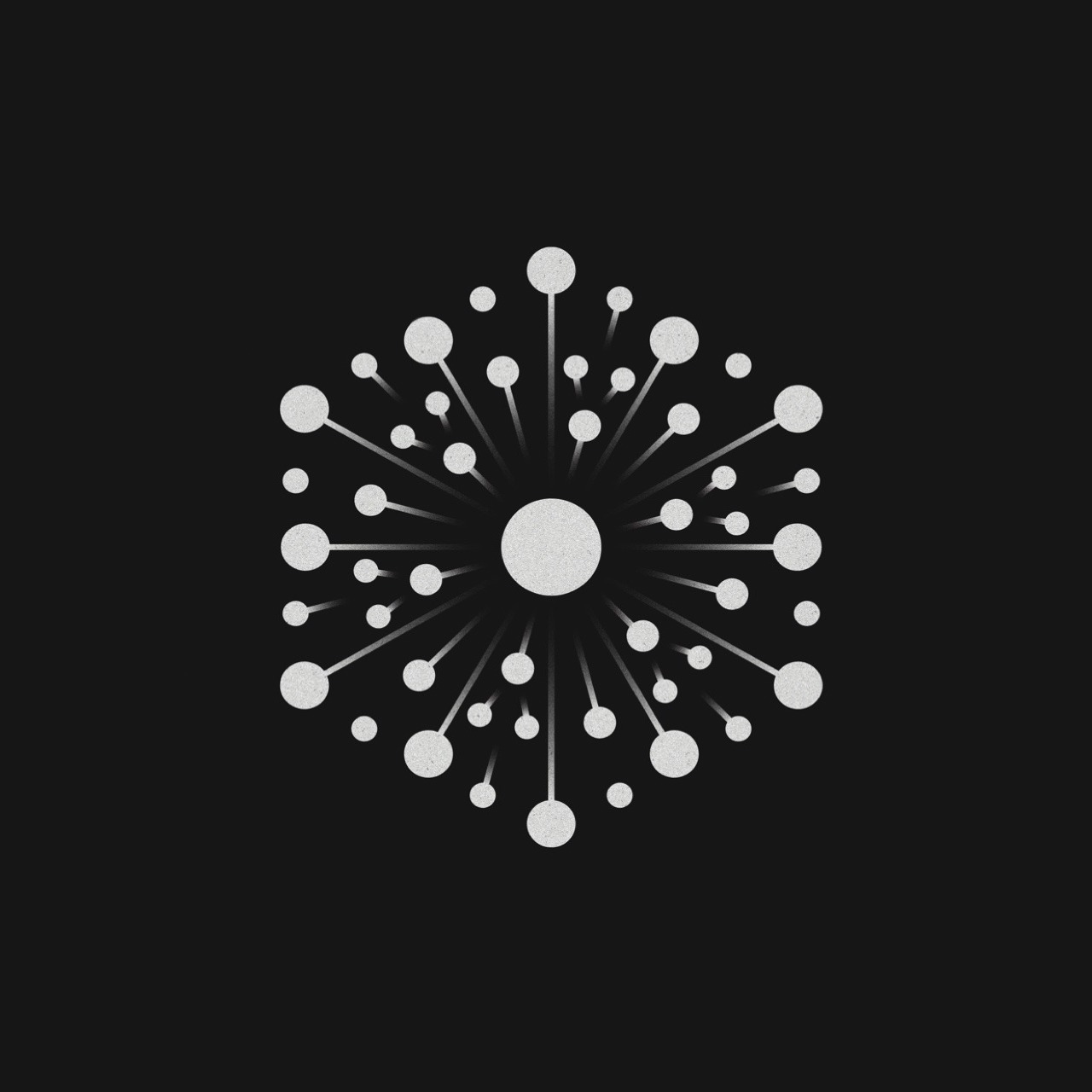
Principal Neighbourhood Aggregation for Graph Nets
Published in
NeurIPS
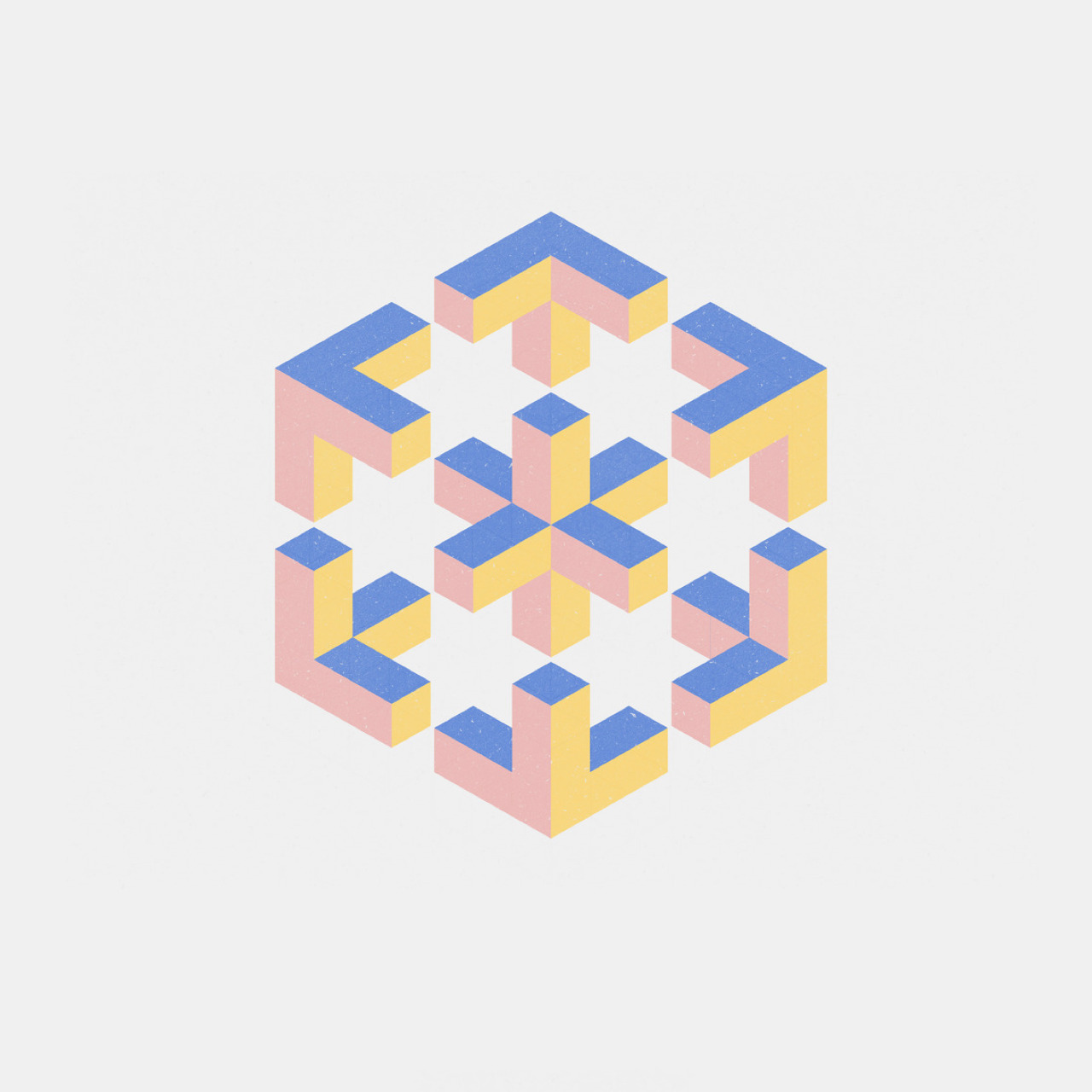
Geodesics in Fibered Latent Spaces
Published in
arXiv
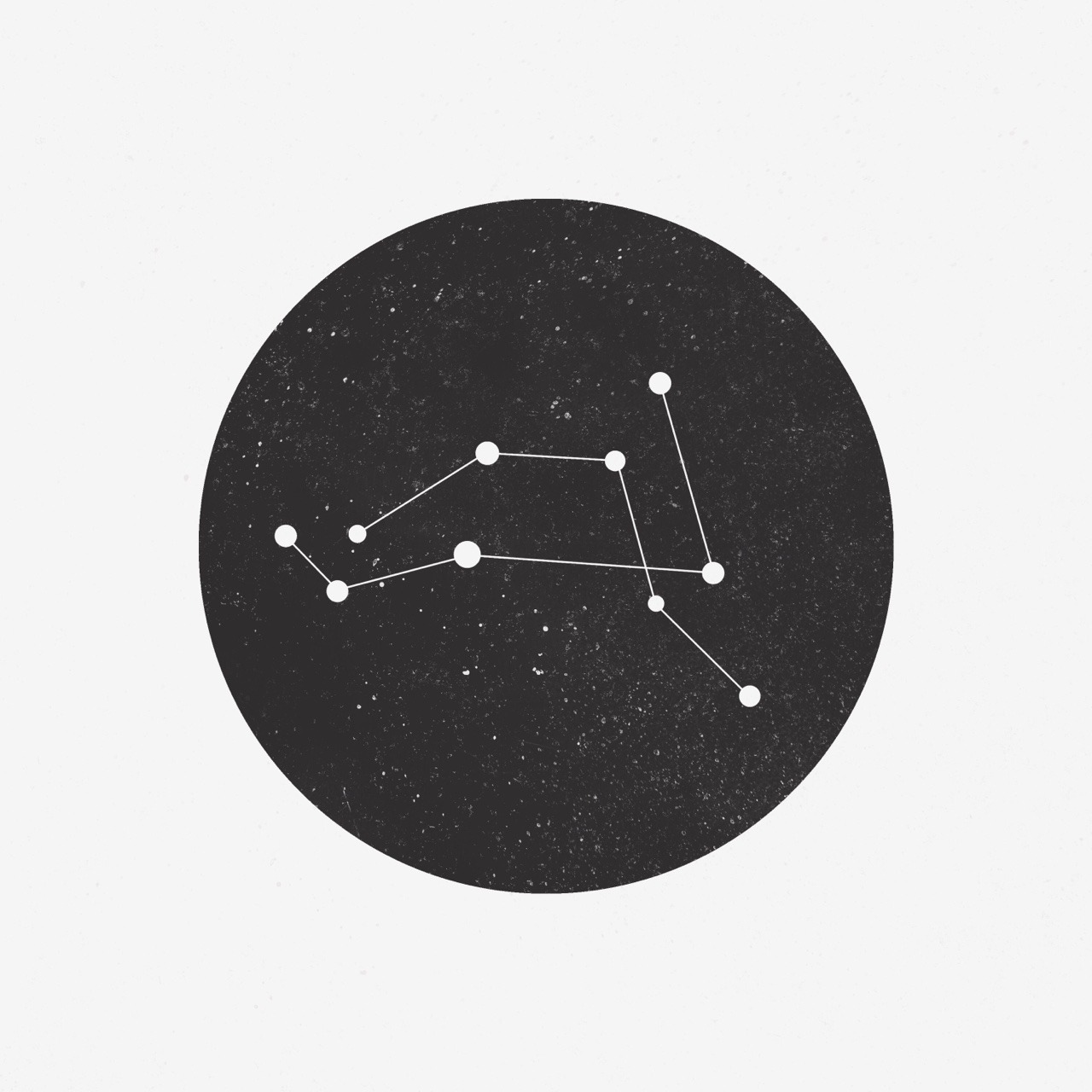
Molecular Design in Synthetically Acessible Chemical Space
Published in
ACS
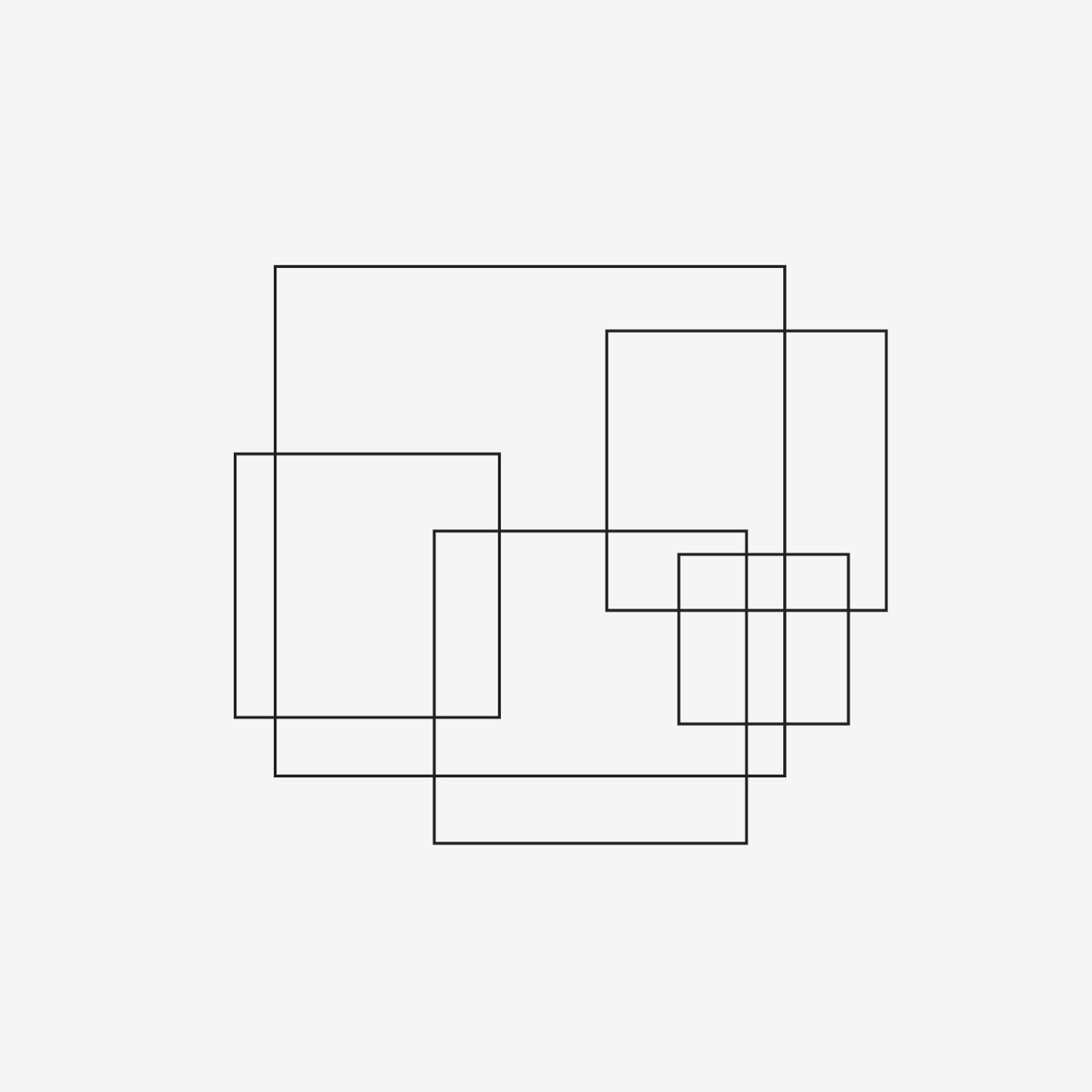
Adaptive Deep Kernel Learning
Published in
arXiv

Interpretable Graph Representation Learning
Published in
arXiv
Interested in Collaborating?
We collaborate with leading researchers around the world and welcome the opportunity to discuss new projects with both academic and industry teams